I am starting today a new series of posts about Apache Kafka (http://kafka.apache.org/). Kafka is an Open Source message broker written in Scala (http://www.scala-lang.org/). Originally it has been developed by LinkedIn (https://ie.linkedin.com/), but then it has been released as Open Source in 2011 and it is currently maintained by the Apache Software Foundation (http://www.apache.org/). Why one should prefer Kafka to a traditional JMS message broker? Here's a short list of convincing reasons:
Kafka uses ZooKeeper (https://zookeeper.apache.org/). The Kafka binaries provide it, so if the hosting machines don't have ZooKeeper on board you can use the one bundled with Kakfa.
The communication between clients and servers happens using a high performant and language agnostic TCP protocol.
Kafka provides APIs to implement producers and consumers using different programming languages. In this series I will focus mainly on Java.
- It's fast: a single Kafka broker running on commodity hardware can handle hundreds of megabytes of reads and writes per second from thousands of clients.
- Great scalability: it can be easily and transparently expanded without downtime.
- Durability and Replication: messages are persisted on disk and replicated within the cluster to prevent data loss (setting a proper configuration using the high number of available configuration parameters you could achieve zero data loss).
- Performances: each broker can handle terabytes of messages without performance impact.
- It allows real time stream processing.
- It can be easily integrated with other popular systems for Big Data architectures like Hadoop, Spark and Storm (later posts of this series will cover the integration with them).
Basic core concepts
These are the core concepts of Kafka you need to get familiar with:- Topics: they are categories or feed names to which upcoming messages are published.
- Producers: any entity that publish messages to a topic.
- Consumers: any entity that subscribes to topics and consumes messages from them.
- Brokers: services that handle read and write operations.
Architecture
And here's the architectural diagram of a typical Kafka cluster: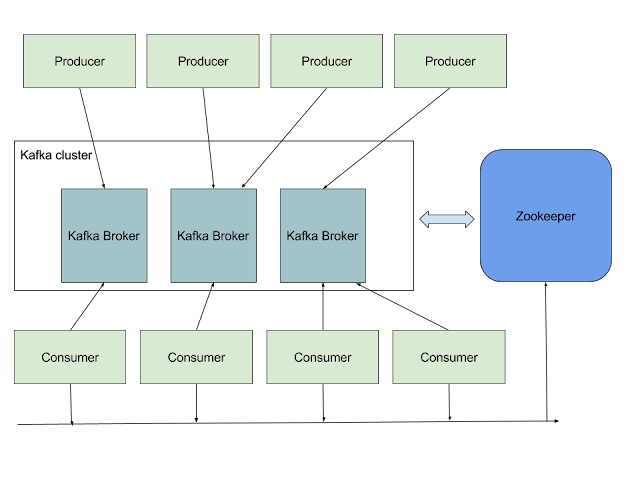
The communication between clients and servers happens using a high performant and language agnostic TCP protocol.
Kafka provides APIs to implement producers and consumers using different programming languages. In this series I will focus mainly on Java.
Use Cases
There are several use cases for Kafka. Here I am going to show a short list, but the scenarios could be much more:- Messaging
- Stream processing
- Log Aggregation
- Metrics
- Web activities tracking
- Event Sourcing
Comments
Post a Comment